Abdul Bari Algorithms - Recurrence Relation and Master's Theorem for Subtracting Functions
Discusses the recurrence relation, which is a mathematical notation to describe a sequence of values based on the previous term, which is useful for describing recursion and time complexity.
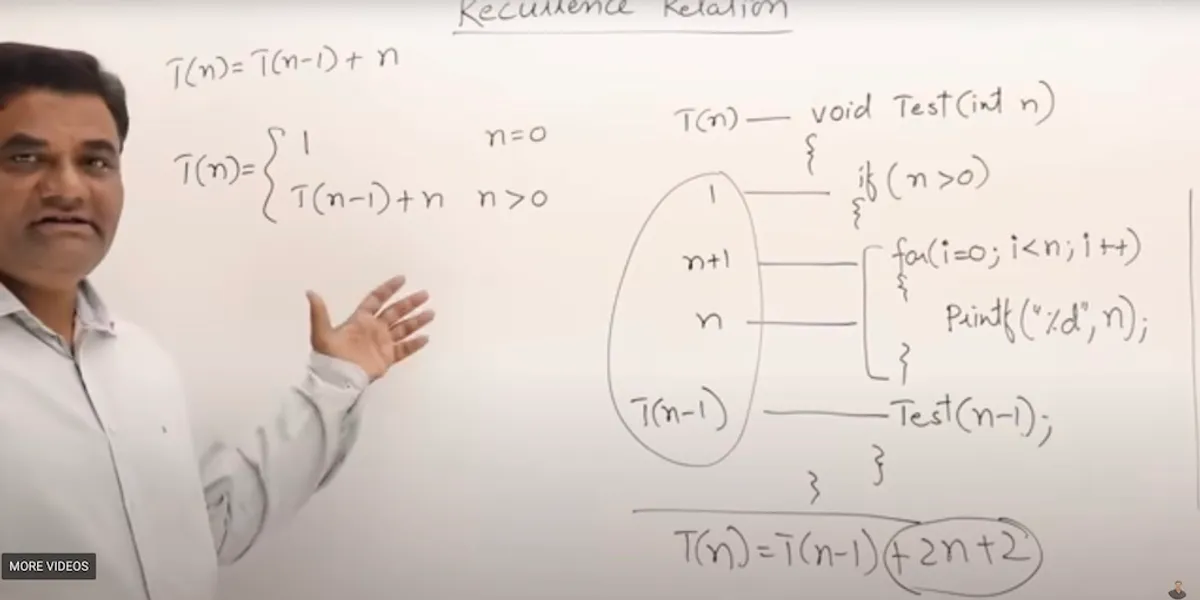
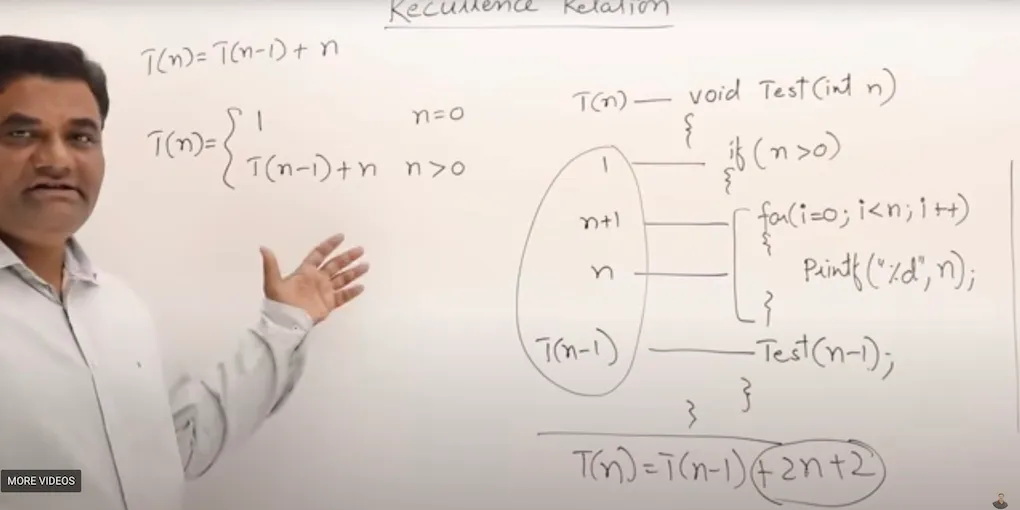
Divide and Conquer
If a problem is large, divide the problem into subproblems, solve them, then recombine the solutions
The subproblems should be the same type of problems, for example, if the main problem is sorting, then the subproblems are sorting
Examples of Divide and Conquer Problems
Binary search
Finding Maximum and Minimum
Merge Sort
Quick Sort
Strassen’s Matrix Multiplication
Subtraction Recurrence Relation 1
Recursive function example
function test(n){
if (n> 0) {
console.log(n);
test(n-1);
}
}
How many times is this function called?
test(3)
-> prints “3”
test(2)
-> prints “2”
test(1)
-> prints “1”
test(0)
-> does not print, or call itself again, since n is not greater than 0
Does work (printing) 3 times, but calls itself 4 times, where it doesn’t print on the last time
If printing is one unit of time, then this takes 3 units of time
So if you pass n
it will make n+1
calls, and print n
times
The time depends on the number of calls, so time complexity is
How do we find the recurrence relation?
1 & \text{when } n=1 \\\ T(n-1)+1 & \text{when } n > 0 \end{cases}$$ #### $T(n)$ based on $T(n-1)$ The original equation We know $T(n)=T(n-1)+1$ We can find $T(n)$ if we know $T(n-1)$ How do we find $T(n-1)$? #### $T(n)$ based on $T(n-2)$ Let's substitute $n-1$ into the equation for $T(n)$ Original equation $T(n)=T(n-1)+1$ Substitute $n-1$ in place of $n$ in the original equation for $T(n)$ $T(n-1)=T((n-1)-1)+1$ This simplifies to $T(n-1)=T(n-2)+1$ Now we can substitute $T(n-1)$ into the original equation for $T(n)$ $T(n) = [T(n-2)+1] +1$ This simplifies to $T(n) = T(n-2) +2$ So now $T(n)$ is in terms of $T(n-2)$ instead of being based on $T(n-1)$ #### $T(n)$ based on $T(n-3)$ We can keep doing this What about $T(n)$ in terms of $T(n-3)$? Substitute $n-2$ into original equation $T(n-2) = T((n-2) -1) + 1$ This simplifies to $T(n-2)=T(n-3)+1$ From before, we know how to write $T(n)$ in terms of $T(n-2)$, so we plug it in to find $T(n)$ in terms of $T(n-3)$ Previous equation: $T(n)=T(n-2)+2 Plug in $T(n-2)$ based on $T(n-3)$ $T(n) = [T(n-3) +1] + 2$ Simplifies to $T(n)=T(n-3)+3$ What if we wanted to continue for $k$ times? What is the smallest value we know, $T(0)=1$ Assume $n-k=0$ Therefore $n=k$ So when $(n-k)=0$ we can write the equation in terms of just $n$ $T(n)=T(n-n)+n$ Simplifies to $T(n)=T(0)+n$ We know $T(0)=1$ So $T(n)=1+n$ ## Subtraction Recurrence Relation 2 {{< youtube IawM82BQ4II >}} ```js function test(n){ if (n>0){ for (let i=0; i< n; i++){ console.log(n); } test(n-1); } } ``` Recurrence relation is $T(n) = T(n-1) + n$ $$T(n) = \begin{cases} 1 & \text{when } n=1 \\\ T(n-1)+n & \text{when } n > 0 \end{cases}$$ For each iteration, it takes $n$ units of time, then calls itself -1 ### Tree Method  We can see the amount of work is $n + (n-1) + (n-2) ... + 1$ To find the total amount of work we can use the [[integer-sum-formula]] $$\sum_{i=1}^n i = \frac{n(n + 1)}{2}$$ This simplifies to $O(n^2)$ for measuring time complexity ### Substitution Method Original equation is $T(n) = T(n-1) + n$ Plug in $n-1$ into original equation $T$ $T(n-1)=T(n-2)+n-1$ Now we substitute this into the original equation to find $T(n)$ in terms of $T(n-2)$ instead of in terms of $T(n-1)$ $T(n)=[T(n-2)+n-1]+n$ Plug Plug in $n-2$ into original equation $T$ $T(n-2) = T(n-3) + n-2$ $T(n)=[T(n-3) + n-2] + (n-1) + n$ Simplify to $T(n) = T(n-3) + (n-2) + (n-1) + n$ If we continue this for $k$ times $T(n) = T(n-k) + (n - (k-1)) + (n - (k-2)) + ... + (n-1) + n$ Assume $n-k=0$ therefore $n=k$ Substitute $n$ for $k$ $T(n)=T(n-n) + T(n-n+1)+(n-n+2) + ... + (n-1) +n$ Simplify further $T(n)=T(0) + 1 + 2 + 3 +... + (n-1) + n$ We can see $1 + 2 + 3 + (n-1) +n$ is the sum of natural numbers, which we can use the [[integer-sum-formula]] to solve $$\sum_{i=1}^n i = \frac{n(n + 1)}{2}$$ And we know $T(0) =1$ So the answer is $1 + \frac{n(n + 1)}{2}$ Which simplifies to $O(n^2)$ in Big O notation ## Subtraction Recurrence Relation 3 {{< youtube MhT7XmxhaCE >}} ```js function test(n){ if (n>0){ for (let i=1; i< n; i=i*2){ console.log(n); } test(n-1); } } ``` We know `(let i=1; i< n; i=i*2)` will execute $log(n)$ times $$T(n) = \begin{cases} 1 & \text{when } n=0 \\\ T(n-1)+ log(n) & \text{when } n > 0 \end{cases}$$ ### Tree Method  Amount of work is $log(n) + log(n-1) + ... + log(2) + log(1)$ $log[n*(n-1) * log(n-2) ... + log(2) + log(1)]$ $log(n!)$ -> log n factorial Equivalent to $O(nlog(n))$ ### Substitution method $T(n)=T(n-1) + log(n)$ Plug in n-2 $T(n)=[T(n-2)+log(n-1)] + log(n)$ $T(n)= [T(n-3)+log(n-2)]+log(n-1) + log(n)$ Assume $n-k=0$, therefore $n=k$ $T(n)=T(n-k) + log(1) + log(2) + ... + log(n-1) + log(n)$ Simplifies to $T(n) = T(0) + log(n!)$ $T(n) = 1 + log(n!)$ $O(n log(n))$ ### Directly Get Answer $T(n)=T(n-1) + 1$ -> $O(n)$ $T(n) = T(n-1) + n$ -> $O(n^2)$ $T(n) = T(n-1) + log(n)$ -> $O(nlog(n))$ $T(n) = T(n-1) + n^2$ -> $O(n^3)$ Just multiply the term after the + by n, since you know it will be repeated n times via recursion What if it's not decreasing by 1? It still works $T(n) = T(n-2) + 1$ -> $n/2$ -> $O(n)$ $T(n) = T(n-100) + n$ -> $O(n^2)$ However, if there's a coeffecient on the function ,it's different though. $T(n) = 2*T(n-1) +1$ ## Subtraction Recurrence Relation 4 ```js function test(n){ if (n>0){ console.log(n); test(n-1); test(n-1); } } ``` $T(n)=2T(n-1)+1$ $$T(n) = \begin{cases} 1 & \text{when } n=0 \\\ 2T(n-1)+1 & \text{when } n > 0 \end{cases}$$ ### Tree Method  function called twice in first row 4 times in second row 8 times in third row So the work done in each row is $2^k$ $1 + 2 + 2^2 + 2^3 + ... + 2^k = n^{k+1}-1$ $$a + ar + ar^2 + ar^3 + ... + ar^k = \frac{a(r^{k+1}-1)}{r-1}$$ In the series above, $a=1$ and $r=2$ So we can use the formula above to find the answer for our tree $$ \frac{1(2^{k+1}-1)}{2-1} $$ Simplifies to $2^{k+1}-1$ Assume $n-k=0$, so $n=k$ $2^{n+1}-1$ So Big O is $O(2^n)$ ### Subsitution Method $T(n)=2T(n-1) + 1$ $T(n)=2[2T(n-2) +1] + 1$ $T(n) = 2^2T(n-2) + 2 + 1$ $T(n)=2^2[2T(n-3) +1] + 2 + 1$ $T(n) = 2^3 T(n-3) + 2^2 + 2 + 1$ $T(n)= 2^kT(n-k) + 2^{k-1}+2^{k-2} + ... + 2^2 + 2 + 1$ Assume $n-k=0$ $n=k$ $T(n) = 2^n T(0) + 1 + 2 + 2^2 + ... + 2^{k-1}$ $T(n) = 2^n * 1 + 2^k -1$ $T(n) = 2^n + 2^n -1$ $T(n) = 2^{n+1}-1$ $O(2^n)$ ## Master's Theorem for Subtracting Functions {{< youtube OynWkEj0S-s >}} $T(n)=T(n-1) + 1$ -> $O(n)$ $T(n) = T(n-1) + n$ -> $O(n^2)$ $T(n) = T(n-1) + log(n)$ -> $O(nlog(n))$ $T(n) = 2T(n-1) + 1$ -> $O(2^n)$ $T(n) = 3T(n-1) + 1$ -> $O(3^n)$ $T(n) = 2T(n-1) + n$ -> $O(n * 3^n)$ ### Master's Theorem General form of recurrence relation $T(n)=aT(n-b)+f(n)$ Assume $a>0$ $b > 0$ $f(n)=O(n^k)$ where $k ≥ 0$ #### Case 1 $a=1$ For example $T(n)=T(n-1) + 1$ Then $O(n^{k+1})$ also can be thought of as $O(n*f(n))$ #### Case 2 $a>1$ For example $T(n) = 2T(n-1) + 1$ Then $O(n^k * a^n)$ #### Case 3 $a>1$ If you're decreasing by more than 1, for example $T(n) = 2T(n-2) + 1$ Then $O(n^k * a^{\frac{n}{b}})$ What if $a<1$ for example .5 Then $O(n^k)$ or $O(f(n))$